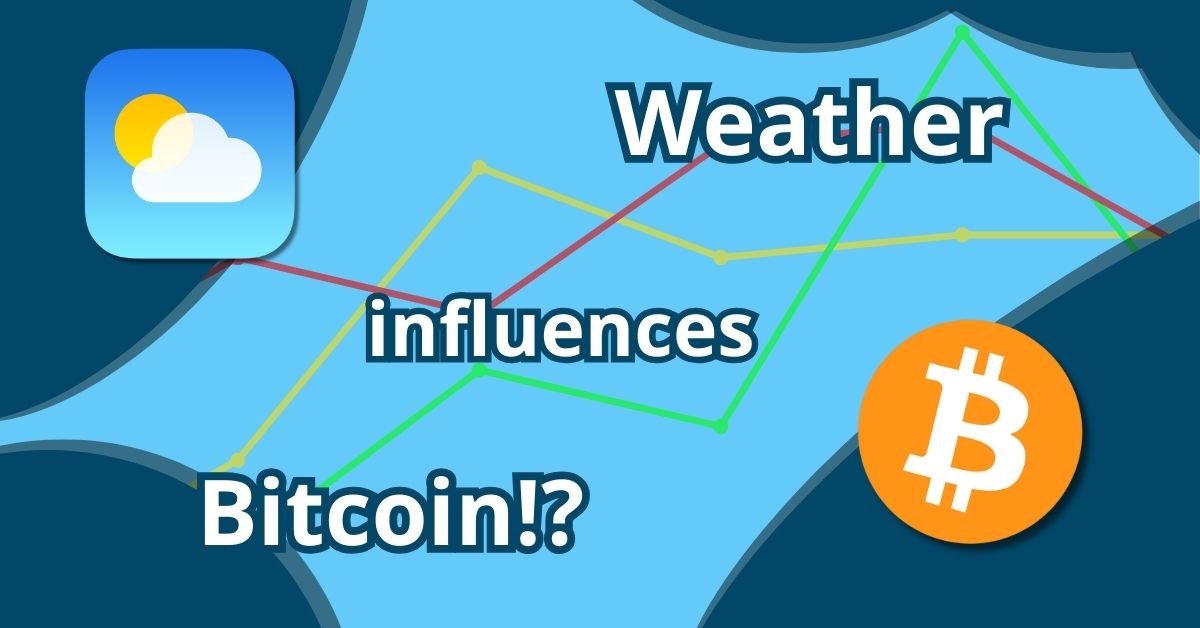
Blog
Our latest articles about data science, machine learning, data analysis and programming. Enjoy!
- Sep 30 2024 · Adam Szulc
- Sep 27 2024 · Maciej Małachowski
TabNet vs XGBoost
Comparison of TabNet and XGBoost on tabular data across binary classification, multiclass classification, and regression tasks. Explore default vs. tuned results and discover TabNet's surprising strengths in regression.
- Sep 27 2024 · Tomasz Marchela, Szymon Niewiński
4 Effective Ways to Visualize LightGBM Trees
"LightGBM is a fast, efficient gradient boosting framework. In this article I show you how to visualize its decision trees using tools like plot_tree for basic plots, dtreeviz for enhanced visuals, graphviz for detailed rendering, and SuperTree for interactive exploration of complex tree structures."
- Sep 27 2024 · Tomasz Marchela, Szymon Niewiński
4 Effective Ways to Visualize Random Forest
"A Random Forest is a supervised machine learning algorithm used for classification and regression. This article demonstrates four ways to visualize Random Forests in Python, including feature importance plots, individual tree visualization using plot_tree, and SuperTree."
- Sep 27 2024 · Tomasz Marchela, Szymon Niewiński
4 Effective Ways to Visualize XGBoost Trees
"XGBoost is a supervised machine learning algorithm used for both classification and regression tasks. This article demonstrates four ways to visualize XGBoost models in Python, including feature importance plots, individual tree visualization using plot_tree, dtreeviz, graphviz, and SuperTree."
- Sep 25 2024 · Maciej Małachowski
Machine Learning Algorithm Comparison
Explore how different machine learning algorithms stack up against each other. This article compares key methods, highlights their strengths and weaknesses, and helps you choose the best one for your needs.
- Sep 16 2024 · Adam Szulc
Transfer data from Postgresql to Google Sheets
Convert your PostgreSQL data into Google Sheets spreadsheet easily using MLJAR studio and Python. Share spreadsheets with other users without leaving your editor
- Sep 13 2024 · Karol Falkowski
Create Retrieval-Augmented Generation using OpenAI API
Unlock the power of Retrieval-Augmented Generation to supercharge your workflow. Learn how to build a simple RAG system using OpenAI models in Python and enhance your AI’s performance with smarter, more accurate results.
- May 15 2024 · Piotr Płoński, Aleksandra Płońska
ChatGPT can talk with all my Python notebooks
We make Python notebooks accessible with REST API. Custom ChatGPT can use actions to execute Python notebooks. You can easily turn any Python code into API available in the ChatGPT
- Apr 10 2024 · Karol Falkowski, Aleksandra Płoński
How to create Invoice Generator in Python
Let us introduce you Invoice Generator, which was created only with Mercury's widgets in Python.