MLJAR AutoML
We’re on our way to revolutionizing the field of machine learning and data analysis. Whether you're a small startup or a large enterprise, MLJAR is here to support you on your machine learning journey.
Data Science Tools
What is AutoML?
The most advanced Machine Learning tool with the simplest User Interface
Open-source MIT license
The Over 100 citations in research papers
More than 1 million downloads
Works with Python with versions 3.8, 3.9, 3.10, 3.11
Uniqe features: automated documentation, fairness metric
Variety of algorithms as: ensemble, stacked ensemble, XGBoost, CatBoost and more
A few steps to achieve your goals!
Define your goals
Clearly outline your analytics goals while specifying how machine learning algorithms can contribute to achieving those objectives.
Provide a good data set & implement MLJAR
Prepare your data for machine learning. Valuable data set is the key to achieve profitable results. Then install mljar-supervised, and let the magic begin!
Deploy models and visualize
Deploy your results or use Mercury. Use the wide visualize libraries to make your work attractive for non-technical users.
Work is done! Coffee time :)
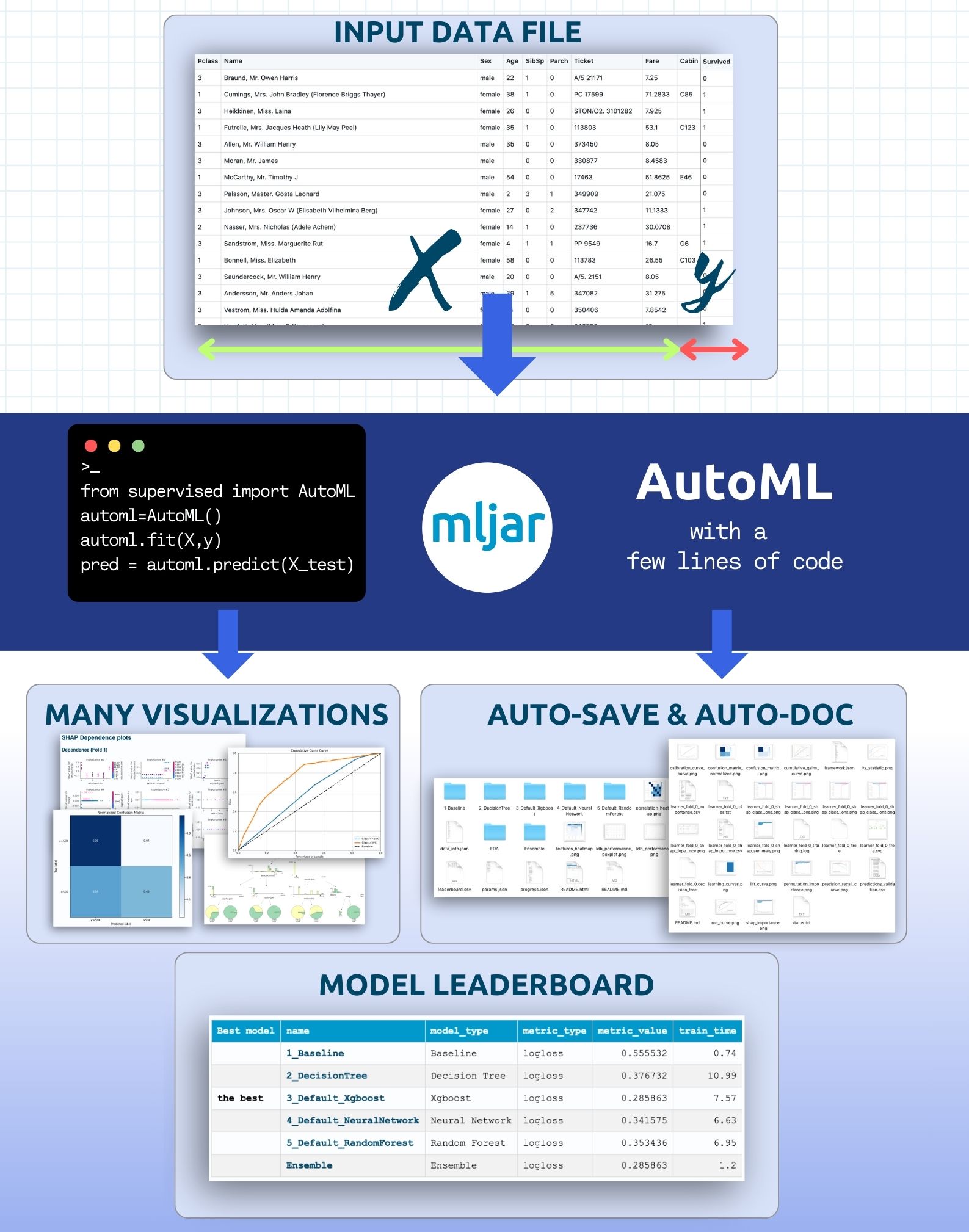
Downloads
Stars
Forks
Users
Features preprocessing
Features selection
Algorithm selection
Golden features
Models ensembling
ML explainability
Features
Discover the unique features that make our AutoML the state of the art. Find those that make your ML project advanced, super easy, and understandable.
Complete pipeline
Simplifies the entire machine learning process from data preprocessing to model deployment.
Golden feature
Efficiently pinpoints the most influential variables for optimal model performance.
Model leaderboard
Enables easy comparison and selection of models based on performance metrics.
Automated reports
The report from running AutoML will contain the table with information about each model score and the time needed to train the model.
Feature selection
MLJAR AutoML takes care of features preprocessing like missing values imputation and converting categoricals, it can also handle target values preprocessing.
Auto-saving models
All models in the AutoML are saved and loaded automatically. No need to call save() or load().
Hyperparameter tuning
Optimizes model performance by automatically searching for the best combination of hyperparameters, saving time and effort.
Variety of algorithms
Choose from many algorithms such as: XGBoost, CatBoost, Neural Networks, Decision trees, Random forest and many more...
Modes
Your comfort using our product is top priority. That is why we created 4 modes instead making you change settings all the time.
Explain
• 75%/25% train/test split
• Explanations
Perform
• 5 fold cross-validation
• Feature engineering
• Search for a model under constraint for prediction time on a single sample
Compete
• Adjusted validation
• Train/test on 5 or 10 fold cross-validation
• Feature engineering
• Try many models
Optuna
• Tune algorithm with Optuna Framework
Discover more.
Find details on our GitHub and in our documentation.