Tensorflow vs Scikit-learn
Have you ever wonder what is the difference between Tensorflow and Sckit-learn? Which one is better? Have you ever needed Tensorflow when you already use Scikit-learn?
Both packages are from the Machine Learning world. The Tensorflow is a library for differentiable programming. It allows constructing Machine Learning algorithms such as Neural Networks. It is used in Deep Learning. It was developed by Google. At the time of writing, its GitHub repository has 149k
stars.
The Scikit-learn is a library that contains ready algorithms for Machine Learning, which can be used to solve tasks like: classification, regression, clustering. It has also a set of methods for data preparation. It was designed to cooperate with packages like NumPy, SciPy, Pandas, Matplotlib. Its GitHub repository has 42.5k
stars.
The Tensorflow was designed to construct Deep Neural Networks which can work with various data formats: tabular data, images, text, audio, videos. On the other hand, the Scikit-learn is rather for the tabular data.
Multi Layer Perceptron
In the case of tabular data, a popular architecture of Neural Network (NN) is a Multi-Layer Perceptron (MLP). In Tensorflow you can, of course, build almost any type of NN. The interesting fact is that the MLP algorithm is also available in Scikit-learn. There are available two algorithms:
- for classification: MLPClassifier
- for regression: MLPRegressor
Let's compare them with Tensorflow! :)
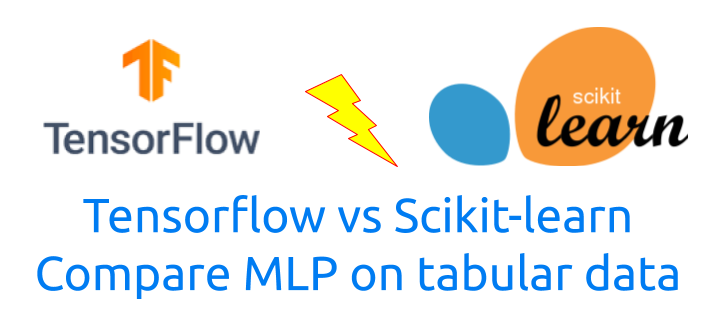
The implmentation of MLP Neural Network with Keras and Tensorflow
In the comparison, I will use simple MLP architecture with 2 hidden layers and Adam optimizer.
To use Tensorflow, I will use Keras which provides higher-level API abstraction with ready NN layers.
The code to construct the MLP with Tensorflow and Keras (TF version == 2.2.0, Keras version == 2.3.1):
# data iformation
input_dim = 12 # number of input features
number_of_classes = 3 # number of classes
ml_task = "multiclass_classification" # task that we are going to solve
# initial hyperparameters
dense_1_size = 32
dense_2_size = 16
learning_rate = 0.05
# define model architecture
model = Sequential()
model.add(Dense(dense_1_size, activation="relu", input_dim=input_dim))
model.add(Dense(dense_2_size, activation="relu"))
if ml_task == "multiclass_classification":
model.add(Dense(number_of_classes, activation="softmax"))
elif ml_task == "binary_classification":
model.add(Dense(1, activation="sigmoid"))
else: # regression
model.add(Dense(1))
# compile the model
opt = Adam(learning_rate=learning_rate)
if ml_task == "multiclass_classification":
model.compile(optimizer=opt, loss="categorical_crossentropy")
elif ml_task == "binary_classification":
model.compile(optimizer=opt, loss="binary_crossentropy")
else: # regression
model.compile(optimizer=opt, loss="mean_squared_error")
And the code to train the MLP with early stopping on 10%
of the train data:
# X and y are training data
batch_size = min(200, X.shape[0])
epochs = 500
stratify = y if ml_task != "regression" else None
X_train, X_vald, y_train, y_vald = train_test_split(
X, y, test_size=0.1, shuffle=True, stratify=stratify
)
# set callbacks
es = EarlyStopping(monitor="val_loss", mode="min", verbose=0, patience=10)
mc = ModelCheckpoint(
"best_model.h5",
monitor="val_loss",
mode="min",
verbose=0,
save_best_only=True,
)
model.fit(
X_train,
y_train,
validation_data=(X_vald, y_vald),
batch_size=batch_size,
epochs=500,
verbose=False,
callbacks=[es, mc],
)
The implementation of the MLP Neural Network with Scikit-learn
The Scikit-learn version == 0.23.2. The code to create the model:
# initial hyperparameters
dense_1_size = 32
dense_2_size = 16
learning_rate = 0.05
epochs = 500
# the model
model = MLPClassifier(
hidden_layer_sizes=(dense_1_size, dense_2_size),
activation="relu",
solver="adam",
learning_rate="constant",
learning_rate_init=learning_rate,
early_stopping=True,
max_iter=epochs
)
If you need the MLP for regression just change the MLPClassifier
to MLPRegressor
.
The code to train the model:
# X and y are training data
model.fit(X, y)
That's all! As you can see, you need much more code with Tensorflow+Keras - it is a much flexible library in terms of constructing Neural Networks and that's why you need to define the whole architecture by yourself. The implementation of MLP from Scikit-learn is more like an off-the-shelf algorithm.
Let's take some data!
I get the data for comparison from Penn Machine Learning Benchamrks. They have a lot of example datasets there. I considered only datasets with 1k or more rows. The methodology of comparison was simple:
- take the dataset, split it,
75%
of data is used for training and25%
for testing, - train different Neural Networks architectures, and select the best one (based on 5-fold cross-validation on train data), use the best model to compute predictions on test samples,
- the data preparation (converting categoricals, target scaling in regression) is handled by AutoML mljar-supervised,
- the hyperparameters tuning is handled by AutoML mljar-supervised,
- for classification, I've used
logloss
and for regressionmean squared error
metrics.
I used below set of hyperparameters for both implementations of MLP:
nn_params = {
"dense_1_size": [16, 32, 64],
"dense_2_size": [4, 8, 16, 32],
"learning_rate": [0.01, 0.05, 0.08, 0.1],
}
The AutoML was drawing values of hyperparameters from the defined set. A model was skipped, if the drawn values were repeated. There were trained up to 13 different Neural Networks for each implementation for each dataset.
The classification task
There were used 66
datasets in classification (both binary and multiclass). The results are reported in the table below. The time reported is in seconds - it is a total time used for checking all drawn architectures.
Dataset | nrows | ncols | classes | Sklearn_logloss | TF_logloss | Sklearn_time | TF_time |
---|---|---|---|---|---|---|---|
Epistasis_2_1000atts_0.4H | 1600 | 1000 | 2 | 0.692272 | 0.693278 | 111.71 | 140.36 |
Epistasis_2_20atts_0.1H | 1600 | 20 | 2 | 0.69291 | 0.694732 | 27.42 | 111.56 |
Epistasis_2_20atts_0.4H | 1600 | 20 | 2 | 0.654114 | 0.666128 | 20.41 | 95.89 |
Epistasis_3_20atts_0.2H | 1600 | 20 | 2 | 0.69219 | 0.693055 | 18.5 | 97.85 |
Heterogeneity_50 | 1600 | 20 | 2 | 0.662284 | 0.692669 | 24.51 | 102.42 |
Heterogeneity_75 | 1600 | 20 | 2 | 0.685651 | 0.684641 | 22.04 | 150.61 |
Hill_Valley_with_noise | 1212 | 100 | 2 | 0.701181 | 0.679262 | 23.4 | 183.42 |
Hill_Valley_without_noise | 1212 | 100 | 2 | 0.693029 | 0.693106 | 22.19 | 151.83 |
adult | 48842 | 14 | 2 | 0.31302 | 0.308794 | 225.9 | 529.94 |
agaricus_lepiota | 8145 | 22 | 2 | 0.000476217 | 1.28456e-08 | 74.34 | 1417.37 |
allbp | 3772 | 29 | 3 | 0.105024 | 0.0873737 | 24.18 | 261.19 |
allhyper | 3771 | 29 | 4 | 0.0543183 | 0.0540823 | 40.24 | 239.92 |
allhypo | 3770 | 29 | 3 | 0.15851 | 0.182609 | 36.58 | 300.35 |
allrep | 3772 | 29 | 4 | 0.0965378 | 0.0877159 | 33.73 | 289.45 |
ann_thyroid | 7200 | 21 | 3 | 0.0663306 | 0.0522971 | 118.12 | 281.09 |
car | 1728 | 6 | 4 | 0.0565009 | 0.0270733 | 25.95 | 336.2 |
car_evaluation | 1728 | 21 | 4 | 0.0355119 | 0.0162453 | 26.77 | 364.57 |
chess | 3196 | 36 | 2 | 0.0381836 | 0.0262048 | 36.16 | 433.17 |
churn | 5000 | 20 | 2 | 0.232703 | 0.233059 | 45.63 | 342.09 |
clean2 | 6598 | 168 | 2 | 0.00358971 | 1.72954e-05 | 106.99 | 1065.38 |
cmc | 1473 | 9 | 3 | 0.872536 | 0.865697 | 12.78 | 255.28 |
coil2000 | 9822 | 85 | 2 | 0.206189 | 0.205792 | 103.68 | 394.26 |
connect_4 | 67557 | 42 | 3 | 0.454379 | 0.449654 | 874.97 | 1194.23 |
contraceptive | 1473 | 9 | 3 | 0.872536 | 0.864501 | 13.88 | 284.1 |
credit_g | 1000 | 20 | 2 | 0.54315 | 0.539984 | 19.76 | 310.27 |
dis | 3772 | 29 | 2 | 0.0700903 | 0.0487778 | 27.56 | 325.68 |
dna | 3186 | 180 | 3 | 0.159211 | 0.149867 | 45.03 | 415.93 |
fars | 100968 | 29 | 8 | 0.469888 | 0.470934 | 612.58 | 1017.39 |
flare | 1066 | 10 | 2 | 0.393395 | 0.388351 | 21.64 | 413.38 |
german | 1000 | 20 | 2 | 0.518058 | 0.521267 | 35.91 | 756.61 |
hypothyroid | 3163 | 25 | 2 | 0.0643045 | 0.0698073 | 84.57 | 900.39 |
kr_vs_kp | 3196 | 36 | 2 | 0.048205 | 0.0500323 | 29.96 | 136.31 |
krkopt | 28056 | 6 | 18 | 16.0305 | 17.489 | 322.73 | 438.99 |
led24 | 3200 | 24 | 10 | 0.847339 | 0.852022 | 28.64 | 97.79 |
led7 | 3200 | 7 | 10 | 0.830557 | 0.822725 | 21.63 | 131.69 |
letter | 20000 | 16 | 26 | 20.9291 | 20.7423 | 230.99 | 263.22 |
magic | 19020 | 10 | 2 | 0.310426 | 0.307443 | 172.18 | 270.02 |
mfeat_factors | 2000 | 216 | 10 | 13.1879 | 12.7521 | 40.47 | 134.59 |
mfeat_fourier | 2000 | 76 | 10 | 5.41445 | 6.88417 | 56.89 | 160.14 |
mfeat_karhunen | 2000 | 64 | 10 | 11.1031 | 11.0863 | 30.74 | 308.79 |
mfeat_morphological | 2000 | 6 | 10 | 6.74621 | 8.46319 | 36.51 | 495.13 |
mfeat_pixel | 2000 | 240 | 10 | 0.105948 | 0.130936 | 88.05 | 534.58 |
mfeat_zernike | 2000 | 47 | 10 | 8.05229 | 8.27279 | 45.2 | 273.91 |
mnist | 70000 | 784 | 10 | 0.106536 | 0.115172 | 1844.9 | 2386.88 |
mofn_3_7_10 | 1324 | 10 | 2 | 0.0100037 | 1.38658e-07 | 24.3 | 620.48 |
mushroom | 8124 | 22 | 2 | 0.00113378 | 2.32726e-08 | 66.92 | 1643.03 |
nursery | 12958 | 8 | 4 | 0.00837833 | 0.00787663 | 218.48 | 413.13 |
optdigits | 5620 | 64 | 10 | 0.0614936 | 0.0626042 | 58.47 | 279.3 |
page_blocks | 5473 | 10 | 5 | 0.111967 | 0.109957 | 38.02 | 302.59 |
parity5+5 | 1124 | 10 | 2 | 0.366705 | 0.296488 | 17.58 | 347.32 |
pendigits | 10992 | 16 | 10 | 0.0244666 | 0.0216054 | 85.26 | 346.54 |
phoneme | 5404 | 5 | 2 | 0.304275 | 0.299198 | 47.83 | 437.41 |
poker | 1025010 | 10 | 10 | 0.00452098 | 0.00726474 | 4062.23 | 2916.7 |
ring | 7400 | 20 | 2 | 0.0829392 | 0.0794716 | 90.69 | 614.34 |
satimage | 6435 | 36 | 6 | 0.242709 | 0.22644 | 63.6 | 365.15 |
segmentation | 2310 | 19 | 7 | 0.118182 | 0.110797 | 34.36 | 313.02 |
shuttle | 58000 | 9 | 7 | 0.00396879 | 0.00399241 | 254.09 | 783.73 |
sleep | 105908 | 13 | 5 | 0.63446 | 0.62662 | 412.82 | 1260.44 |
solar_flare_2 | 1066 | 12 | 6 | 0.567718 | 0.549793 | 23.22 | 454.14 |
spambase | 4601 | 57 | 2 | 0.164812 | 0.162715 | 55.17 | 511.57 |
splice | 3188 | 60 | 3 | 0.314676 | 0.365399 | 46.27 | 309.57 |
texture | 5500 | 40 | 11 | 15.8874 | 20.6093 | 58.74 | 573.67 |
twonorm | 7400 | 20 | 2 | 0.0600894 | 0.0581619 | 46.37 | 717.49 |
waveform_21 | 5000 | 21 | 3 | 0.293883 | 0.297824 | 45.55 | 544.52 |
waveform_40 | 5000 | 40 | 3 | 0.290914 | 0.291565 | 47.11 | 536.87 |
wine_quality_red | 1599 | 11 | 6 | 0.930231 | 0.954956 | 34.55 | 437.98 |
The results can be plotted as scatter plot:
Let's zoom to the [0,1]
range:
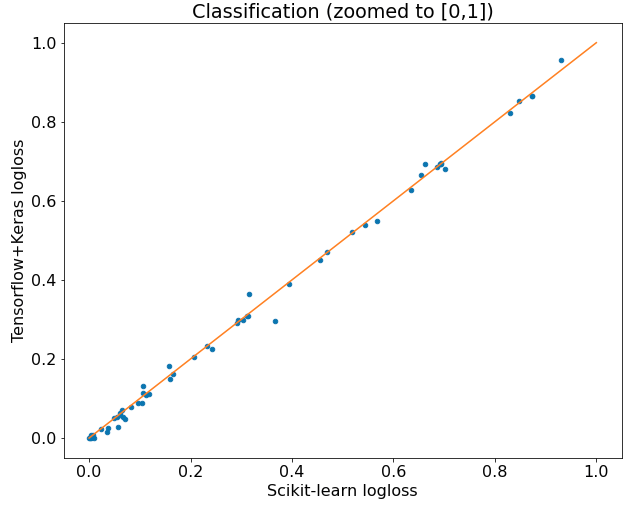
There were 66
datasets and the Tensorflow implementation was 39
times better than Scikit-learn implementation. The differences weren't huge.
It's worth to take a look at times of computation. All computations were on the CPU. The mean time of computation for Scikit-learn was 177
seconds while for Tensorflow it was 508
seconds. The Scikit-learn is much faster. Maybe because TF is intended to be used on GPU rather than CPU? I don't know.
The regression task
There were 48
datasets in the regression task. The results are in the table below:
Dataset | nrows | ncols | Scikit_MSE | TF_MSE | Scikit_time | TF_time |
---|---|---|---|---|---|---|
1028_SWD | 1000 | 10 | 0.351288 | 0.350476 | 16.9 | 84.53 |
1029_LEV | 1000 | 4 | 0.404629 | 0.398672 | 19.1 | 64.97 |
1030_ERA | 1000 | 4 | 2.51881 | 2.5505 | 17.34 | 92.35 |
1191_BNG_pbc | 1000000 | 18 | 688631 | 688662 | 3567.38 | 3557.82 |
1193_BNG_lowbwt | 31104 | 9 | 207709 | 208230 | 135.2 | 224.09 |
1196_BNG_pharynx | 1000000 | 10 | 85241.6 | 85288.7 | 3562.32 | 3290.37 |
1199_BNG_echoMonths | 17496 | 9 | 135.59 | 135.655 | 86.55 | 152.94 |
1201_BNG_breastTumor | 116640 | 9 | 91.819 | 91.538 | 416.2 | 879.4 |
1203_BNG_pwLinear | 177147 | 10 | 7.64677 | 7.64574 | 431.18 | 874.16 |
1595_poker | 1025010 | 10 | 0.0828265 | 0.0928364 | 3371.3 | 3165.36 |
197_cpu_act | 8192 | 21 | 6.04194 | 6.18341 | 102.1 | 197.32 |
201_pol | 15000 | 48 | 6.82901 | 6.484 | 128.53 | 220.26 |
215_2dplanes | 40768 | 10 | 1.00462 | 1.00613 | 149.9 | 395.09 |
218_house_8L | 22784 | 8 | 7.6172e+08 | 7.66826e+08 | 153.39 | 389.73 |
225_puma8NH | 8192 | 8 | 10.2505 | 10.3683 | 39.81 | 255.74 |
227_cpu_small | 8192 | 12 | 7.90558 | 8.14906 | 47.62 | 295.11 |
294_satellite_image | 6435 | 36 | 0.490969 | 0.482076 | 79.77 | 324.38 |
344_mv | 40768 | 10 | 0.00101928 | 0.00076863 | 191.04 | 563.72 |
4544_GeographicalOriginalofMusic | 1059 | 117 | 0.274018 | 0.252322 | 24.36 | 212.47 |
503_wind | 6574 | 14 | 9.51609 | 9.43174 | 35.83 | 337.39 |
529_pollen | 3848 | 4 | 2.00028 | 1.98782 | 20.59 | 320.87 |
537_houses | 20640 | 8 | 2.8563e+09 | 2.78972e+09 | 130.66 | 449.33 |
562_cpu_small | 8192 | 12 | 7.90558 | 8.03778 | 46.5 | 391.88 |
564_fried | 40768 | 10 | 1.06119 | 1.04008 | 199.99 | 648.26 |
573_cpu_act | 8192 | 21 | 6.04194 | 6.11835 | 97.13 | 351.69 |
574_house_16H | 22784 | 16 | 9.59662e+08 | 9.6518e+08 | 172.56 | 545.2 |
583_fri_c1_1000_50 | 1000 | 50 | 0.784129 | 0.767178 | 20.21 | 275.6 |
586_fri_c3_1000_25 | 1000 | 25 | 0.515327 | 0.645629 | 18.89 | 435.76 |
588_fri_c4_1000_100 | 1000 | 100 | 0.84392 | 0.917454 | 18.02 | 333.99 |
589_fri_c2_1000_25 | 1000 | 25 | 0.449973 | 0.572697 | 20.68 | 352.22 |
590_fri_c0_1000_50 | 1000 | 50 | 0.341366 | 0.373753 | 23.86 | 358.61 |
592_fri_c4_1000_25 | 1000 | 25 | 0.527293 | 0.618391 | 19.53 | 524.52 |
593_fri_c1_1000_10 | 1000 | 10 | 0.0616339 | 0.106806 | 23.56 | 351.52 |
595_fri_c0_1000_10 | 1000 | 10 | 0.0737259 | 0.0729692 | 15.99 | 353.94 |
598_fri_c0_1000_25 | 1000 | 25 | 0.223486 | 0.234454 | 22.21 | 356.71 |
599_fri_c2_1000_5 | 1000 | 5 | 0.0255253 | 0.0254379 | 23.48 | 376.14 |
606_fri_c2_1000_10 | 1000 | 10 | 0.0528226 | 0.0531012 | 25.89 | 391.02 |
607_fri_c4_1000_50 | 1000 | 50 | 0.792365 | 0.777099 | 20.87 | 560.02 |
608_fri_c3_1000_10 | 1000 | 10 | 0.0529629 | 0.0580307 | 25.5 | 353.62 |
609_fri_c0_1000_5 | 1000 | 5 | 0.0420409 | 0.043429 | 15.71 | 463.82 |
612_fri_c1_1000_5 | 1000 | 5 | 0.0313801 | 0.0311381 | 24.51 | 526.56 |
618_fri_c3_1000_50 | 1000 | 50 | 0.8721 | 0.881979 | 18.3 | 517.28 |
620_fri_c1_1000_25 | 1000 | 25 | 0.713895 | 0.748985 | 15.09 | 370.89 |
622_fri_c2_1000_50 | 1000 | 50 | 0.814633 | 0.876445 | 21.74 | 486.49 |
623_fri_c4_1000_10 | 1000 | 10 | 0.0580734 | 0.059318 | 26.21 | 461.91 |
628_fri_c3_1000_5 | 1000 | 5 | 0.0664999 | 0.0591807 | 19.43 | 465.6 |
banana | 5300 | 2 | 0.287642 | 0.286359 | 39.67 | 741.51 |
titanic | 2201 | 3 | 0.662838 | 0.659142 | 24.62 | 719.5 |
The results can be plotted as scatter plot:
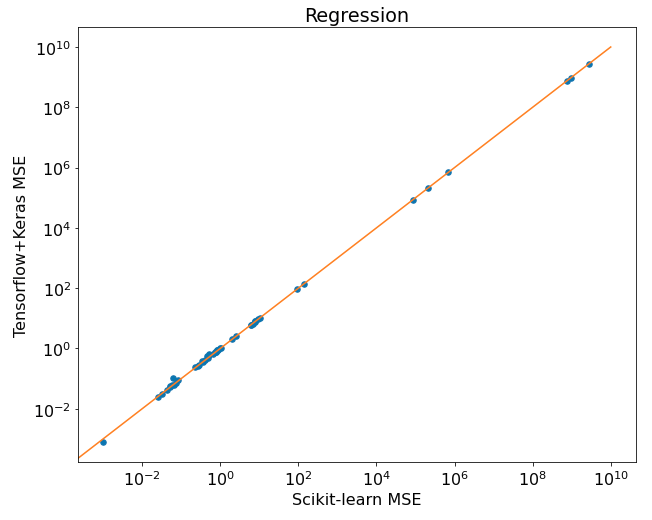
Let's zoom the [0,100]
range:
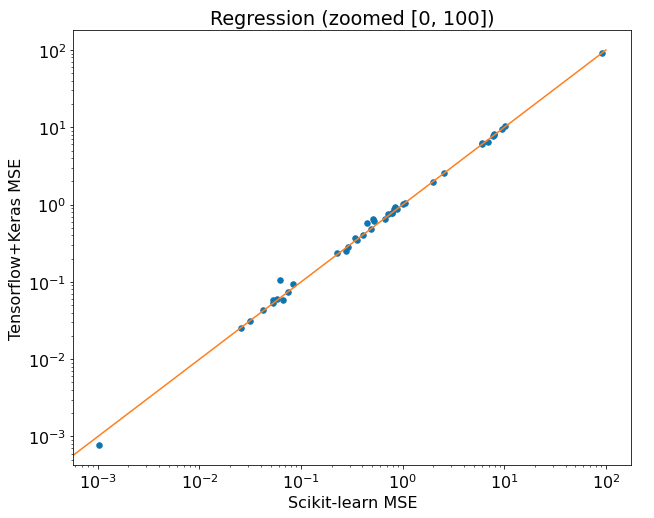
Surprise, surprise! The Scikit-learn MLPRegressor
was 28
times out of 48
datasets better than Tensorflow! Again, as in classification, the differences aren't huge. In time comparison, by average it is 286
seconds for Scikit-learn and 586
seconds for Tensorflow.
Summary
- The Tensorflow library is intended to be used to define Deep Neural Networks. All algorithms are defined by the user manually. The high-level packages as Keras can help to speed up the process of NN construction. The library can be used with a variety of data types: tabular, images, text, audio.
- The Scikit-learn package has ready algorithms to be used for classification, regression, clustering ... It works mainly with tabular data.
- When comparing Tensorflow vs Scikit-learn on tabular data with classic Multi-Layer Perceptron and computations on CPU, the Scikit-learn package works very well. It has similar or better results and is very fast.
- When you are using Scikit-learn and need classic MLP architecture, in my opinion, there is no need to grab Tensorflow (which is, by the way, quite a big package over 500MB on PyPi)
If you are developing Machine Learning models and want to save time, you definitely should try our AutoML mljar-supervised! It is amazing :)